June 29, 2020
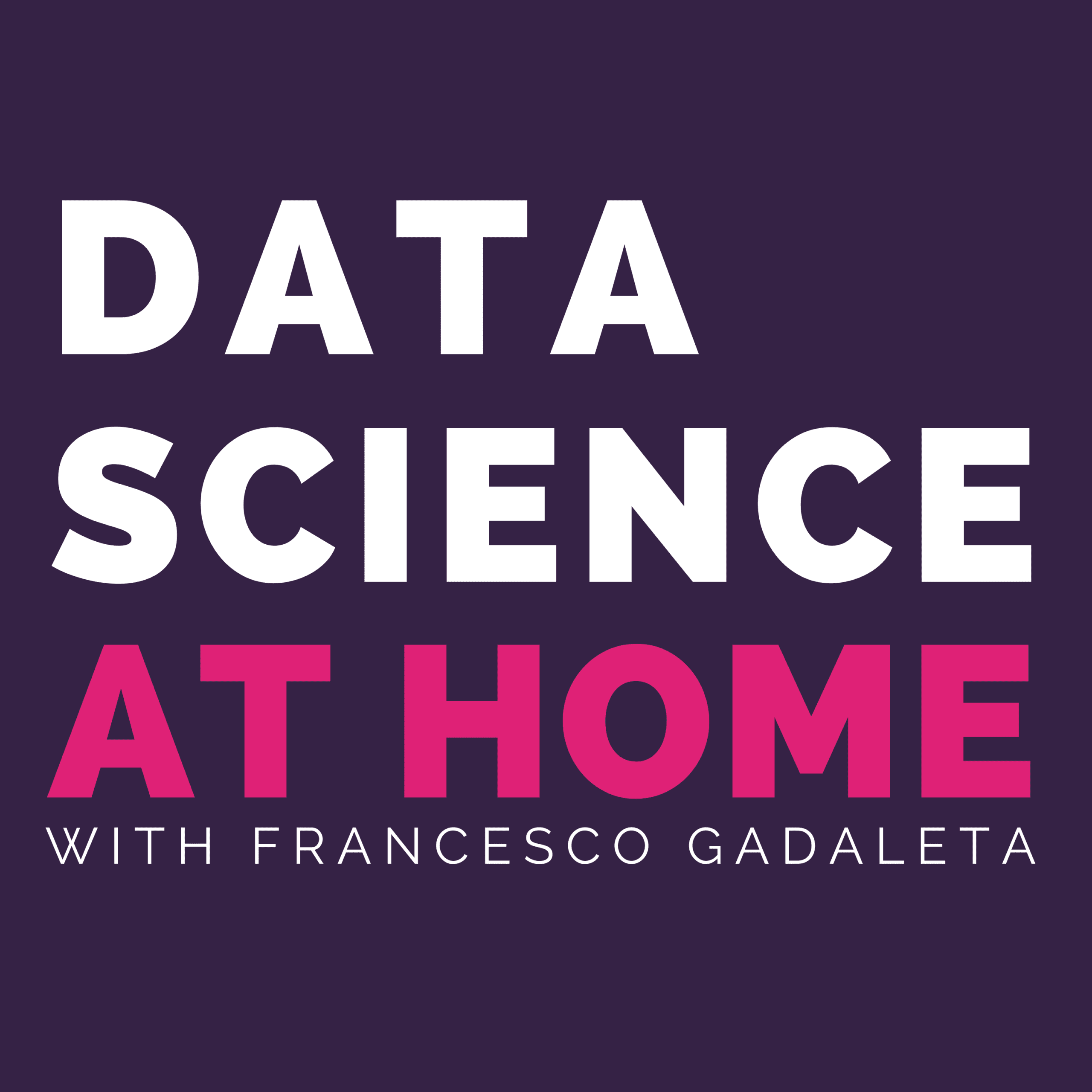
In this episode I make a non exhaustive list of machine learning tools and frameworks, written in Rust. Not all of them are mature enough for production environments. I believe that community effort can change this very quickly.
To make a comparison with...